Generalizing to Unseen Domains with Wasserstein Distributional Robustness under Limited Source Knowledge
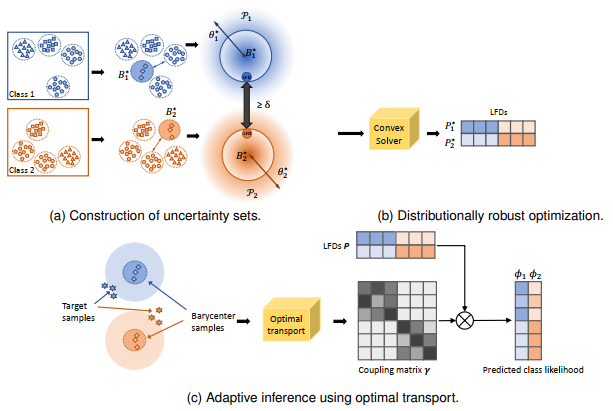
An overview of our WDRDG framework: (a) Wasserstein uncertainty set construction for each class based on the empirical Wasserstein barycenters and radius obtained from given source domains. (b) distributionally robust optimization to solve for the least favorable distributions; (c) adaptive inference for target testing samples.
Domain generalization aims at learning a universal model that performs well on unseen target domains, incorporating knowledge from multiple source domains. In this research, we consider the scenario where different domain shifts occur among conditional distributions of different classes across domains. When labeled samples in the source domains are limited, existing approaches are not sufficiently robust. To address this problem, we propose a novel domain generalization framework called Wasserstein Distributionally Robust Domain Generalization (WDRDG), inspired by the concept of distributionally robust optimization. We encourage robustness over conditional distributions within class-specific Wasserstein uncertainty sets and optimize the worst-case performance of a classifier over these uncertainty sets. We further develop a test-time adaptation module leveraging optimal transport to quantify the relationship between the unseen target domain and source domains to make adaptive inference for target data. Experiments on the Rotated MNIST, PACS and the VLCS datasets demonstrate that our method could effectively balance the robustness and discriminability in challenging generalization scenarios.
Publication
Workshop Paper
In the preliminary version, we proposed Distributional Robust Domain Generalization (DRDG) and tested its effectiveness on image classification tasks.
Jingge Wang, Yang Li*, Yanli Xie and Yao Xie. Class-conditioned Domain Generalization via Wasserstein Distributional Robust Optimization, Robust and Reliable Machine Learning in the Real World Workshop at ICLR, 2021 (Virtual) | ppt |
Journal Paper
In the journal version, we improved the original formulation on balancing between discriminability and robustness. We also introduce the test-time adaptation module that handles class prior distribution shift.
Jingge Wang, Liyan Xie, Yao Xie, Shao-Lun Huang and Yang Li, Generalizing to Unseen Domains with Wasserstein Distributional Robustness under Limited Source Knowledge, in IEEE Journal of Selected Topics in Signal Processing, 14: 8, 2024 | ppt |
@article{wang2024generalizing, title={Generalizing to Unseen Domains with Wasserstein Distributional Robustness under Limited Source Knowledge}, author={Wang, Jingge and Xie, Liyan and Xie, Yao and Huang, Shao-Lun and Li, Yang}, journal={IEEE Journal of Selected Topics in Signal Processing}, volume={14}, number={8}, year={2024} }