Efficient Prediction of Model Transferability in Semantic Segmentation Tasks
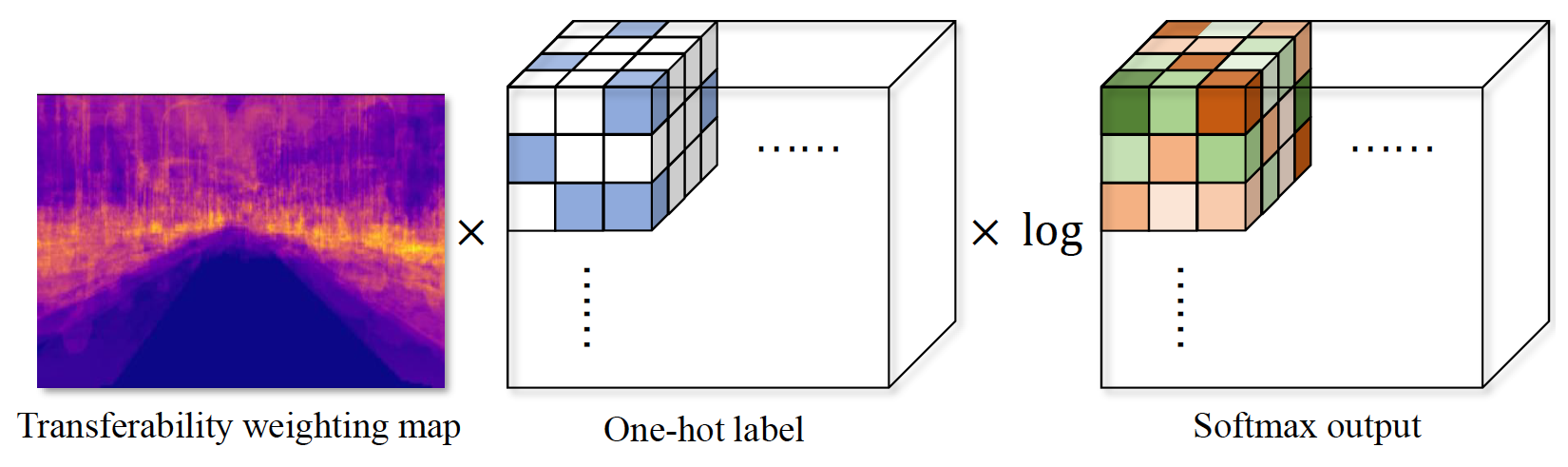
How to efficiently select highly transferable pretrained models remains a challenging problem in few-shot semantic segmentation tasks. Existing transferability metrics for classification tasks are difficult to compute on segmentation data due to the high-dimensional output of the segmentation model. In this work, we generalize existing transferability metrics to efficiently predict the transferability of semantic segmentation models, by calculating transferability scores over the sampled pixel-wise features. Then with the help of transferability, we propose a transferability-weighted finetuning method which puts more importance on those low-transferability regions to improve the overall transfer accuracy on the target task. Experiments on a challenging benchmark show that the transferability scores produced by our adaptation method are highly correlated with the groundtruth transfer accuracy, achieving 0.718 Spearman’s correlation coefficient on average and at least 67× gain on efficiency. In addition, our transferability-weighted finetuning method outperforms vanilla fine-tuning by 4% in transfer accuracy.
Publication
Yang Tan , Yicong Li, Yang Li, and Xiaoping ZHang. Efficient Prediction of Model Transferability in Semantic Segmentation Tasks, 2023 IEEE International Conferenceon Image Processing (ICIP) (Accepted) | ppt |
@inproceedings{icip23, title={Efficient Prediction of Model Transferability in Semantic Segmentation Tasks}, author={Yang Tan , Yicong Li, Yang Li, and Xiaoping ZHang.}, booktitle={2019 IEEE International Conference on Image Processing (ICIP)}, year={2023}, organization={Springer} }