Topology-Preserving Hard Pixel Mining for Tubular Structure Segmentation
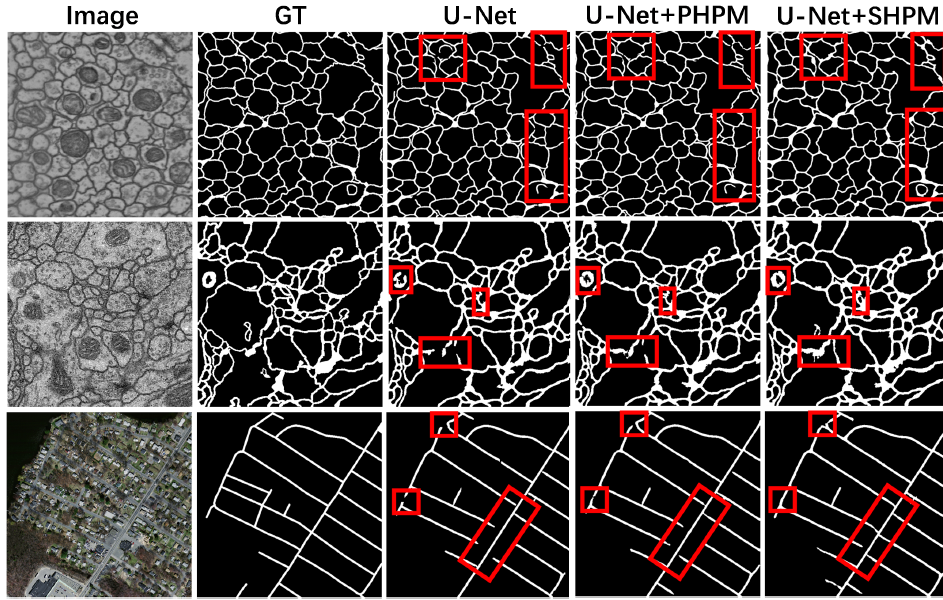
Maintaining accurate topology in image segmentation, especially for tubular structures such as borders of neurons, vessels or roads, is essential in many research fields but remains unsolved. Existing neural segmentation networks are prone to topological errors on these fine-scale structures. We propose a skeleton-based hard pixel mining strategy and incorporate it into training end-to-end segmentation networks. In particular, we penalize the most topology-relevant yet mis-segmented pixels in overall cost function. Skeletal hard pixels are mined through several simple logical and morphological operations which are fast and scalable. We evaluate our method on six public datasets of different domains, including neurons, roads and coronary arteries. Our approach demonstrates training speed comparable to the base model, while achieving a significant reduction in the number of erroneous connected components by 17.14 and loops by 53.87, respectively. Extensive experimental results demonstrate that our method outperforms state-of-the-art computational topology-based method in topological preservation while maintaining competitive volumetric accuracy.
Code and Demo
Publication
Guoqing Zhang, Caixia Dong and Yang Li. Topology-Preserving Hard Pixel Mining for Tubular Structure Segmentation. The 34th British Machine Vision Conference (BMVC), Aberdeen, UK, 2023 (Accepted) | ppt |