Reinforced Domain Selection for Continuous Domain Adaptation
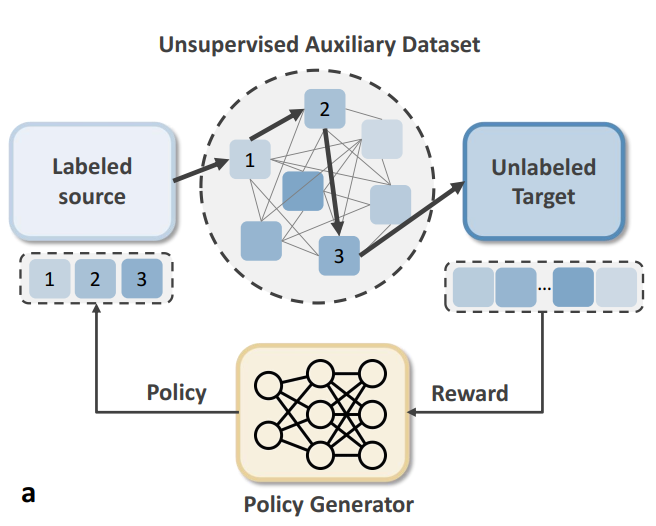
Overview of Continual Domain Adaptation using Reinforcement Learning. Our approach learns a policy generator to select the optimal intermediate domains during continuous domain adaptation.
Continuous Domain Adaptation (CDA) effectively bridges significant domain shifts by progressively adapting from the source domain through intermediate domains to the target domain. However, selecting intermediate domains without explicit metadata remains a substantial challenge that has not been extensively explored in existing studies. To tackle this issue, we propose a novel framework that combines reinforcement learning with feature disentanglement to conduct domain path selection in an unsupervised CDA setting. Our approach introduces an innovative unsupervised reward mechanism that leverages the distances between latent domain embeddings to facilitate the identification of optimal transfer paths. Furthermore, by disentangling features, our method facilitates the calculation of unsupervised rewards using domainspecific features and promotes domain adaptation by aligning domaininvariant features. This integrated strategy is designed to simultaneously optimize transfer paths and target task performance, enhancing the effectiveness of domain adaptation processes. Extensive empirical evaluations on datasets such as Rotated MNIST and ADNI demonstrate substantial improvements in prediction accuracy and domain selection efficiency, establishing our method’s superiority over traditional CDA approaches.
Publication
Hanbing Liu, Huaze Tang, Yanru Wu, Yang Li*, Xiao-Ping Zhang. Reinforced Domain Selection forContinuous Domain Adaptation. In 2025 IEEE International Conference on Acoustics, Speech, andSignal Processing (ICASSP’25). IEEE, 2025 (Accepted) | ppt |
@inproceedings{liu2025reinforced, title={Reinforced Domain Selection for Continuous Domain Adaptation}, author={Liu, Hanbing and Tang, Huaze and Wu, Yanru and Li, Yang and Zhang, Xiao-Ping}, booktitle={2025 IEEE International Conference on Acoustics, Speech, and Signal Processing (ICASSP'25)}, year={2025}, organization={IEEE}, note={Accepted} }