Joint PVL Detection and Manual Ability Classification Using Semi-supervised Multi-task Learning
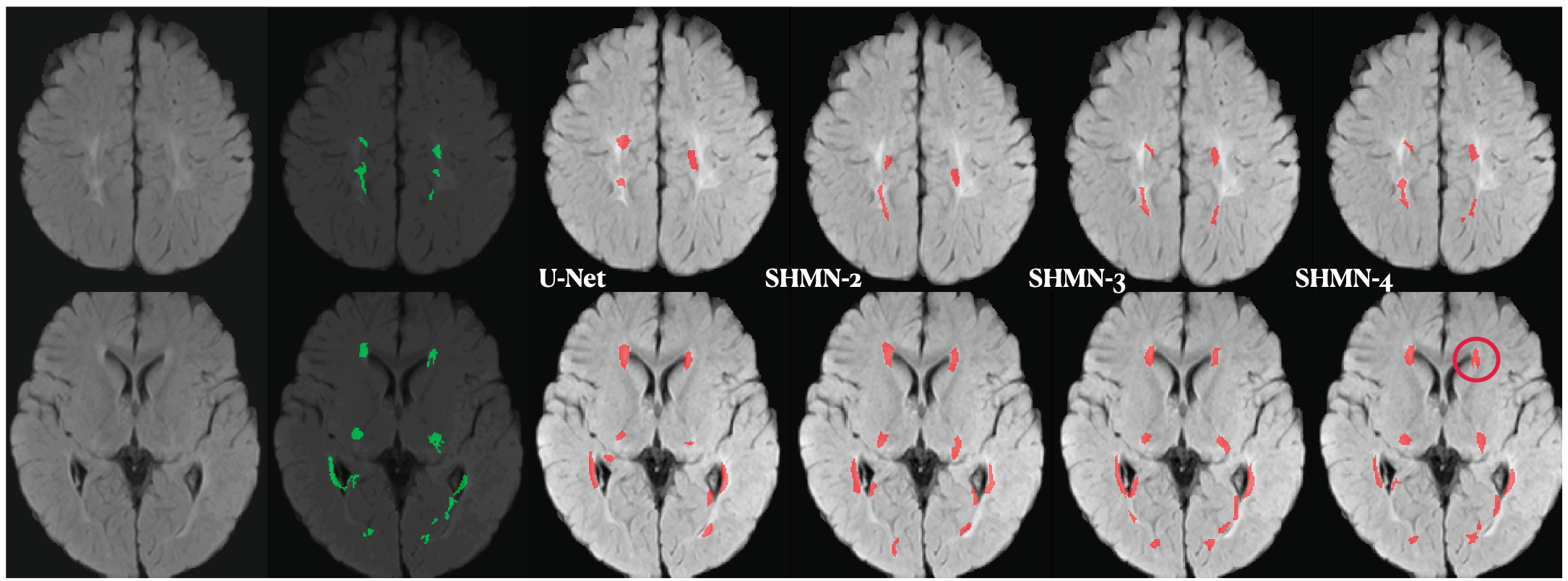
Visual results of the proposed framework (SHMN) and U-Net. From left to right: sample T2 FLAIR slices; expert annotations of PVL lesions in green; segmentation of PVL lesions generated by U-Net and SHMN trained with 2,3,4 tasks) in red.
Among symptoms of cerebral palsy (CP), the degree of hand function impairment in young children is hard to assess due to large inter-personal variability and differences in evaluators’ experience. To help design better treatment strategies, accurate identification and delineation of manual ability injury level is a major clinical concern. Periventricular leukomalacia (PVL), a form of brain lesion in periventriular white matter in premature infants, is a leading cause of CP and have clinical associations with motor function injuries. In this paper, we exploit the correlation between PVL lesion segmentation and manual ability classification (MAC) to improve the identification performance of both tasks for T2 FLAIR MRI scans. Particularly, we propose a semi-supervised multitask learning framework to jointly learn from heterogeneous datasets. Two clinically related auxiliary tasks, lesion localization and ventricle segmentation, are also incorporated to improve the classification accuracy while requiring only a small amount of manual annotations. Using two datasets containing 24 labeled PVL samples and 87 labeledMAC samples, the proposed model significantly outperforms single-task methods, achieving a dice score of 0.607 for PVL lesion segmentation and 84.3% accuracy for manual ability classification.
Publication
Jingyun Yang, Jie Hu, Yicong Li, Heng Liu and Yang Li*. Joint PVL Detection and Manual AbilityClassification Using Semi-supervised Multi-task Learning. In Proceedings of Conference on MedicalImage Computing and Computer-Assisted Intervention (MICCAI), pp. 453-463. Springer, Cham, 2021 | ppt |
@inproceedings{yang2021joint, title={Joint PVL Detection and Manual Ability Classification Using Semi-supervised Multi-task Learning}, author={Yang, Jingyun and Hu, Jie and Li, Yicong and Liu, Heng and Li, Yang}, booktitle={International Conference on Medical Image Computing and Computer-Assisted Intervention}, pages={453--463}, year={2021}, organization={Springer} }