pFedGPA: Diffusion-based generative parameter aggregation for personalized federated learning
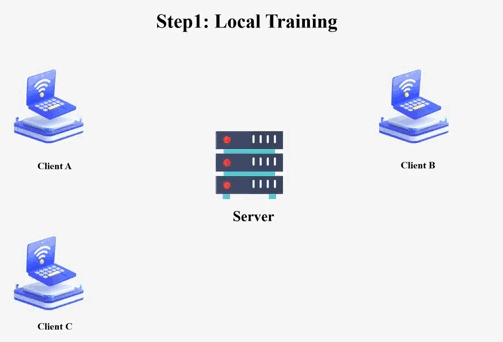
Personalized federated learning framework in pFedGPA: Local models on edge devices are trained independently, and their updated parameters are sent to the central server. The central server aggregates these parameters and generates new parameters for each client model, enabling privacy-preserving personalized distributed learning.
Federated Learning (FL) offers a decentralized approach to model training, where data remains local and only model parameters are shared between the clients and the central server. Traditional methods, such as Federated Averaging (FedAvg), linearly aggregate these parameters which are usually trained on heterogeneous data distributions, potentially overlooking the complex, high-dimensional nature of the parameter space. This can result in degraded performance of the aggregated model. While personalized FL approaches can mitigate the heterogeneous data issue to some extent, the limitation of linear aggregation remains unresolved.
To alleviate this issue, we investigate the generative approach of diffusion model and propose a novel generative parameter aggregation framework for personalized FL, pFedGPA. In this framework, we deploy a diffusion model on the server to integrate the diverse parameter distributions and propose a parameter inversion method to efficiently generate a set of personalized parameters for each client. This inversion method transforms the uploaded parameters into a latent code, which is then aggregated through denoising sampling to produce the final personalized parameters. By encoding the dependence of a client’s model parameters on the specific data distribution using the high-capacity diffusion model, pFedGPA can effectively decouple the complexity of the overall distribution of all clients’ model parameters from the complexity of each individual client’s parameter distribution. Our experimental results consistently demonstrate the superior performance of the proposed method across multiple datasets, surpassing baseline approaches.
Publication
Lai, Jiahao, Jiagi Li, Jian Xu, Yanru Wu, Boshi Tang, Siqi Chen, Yongfeng Huang, Wenbo Ding,and Yang Li, pFedGPA: Diffusion-based generative parameter aggregation for personalized federatedlearning, in Proceedings of the 38th Annual AAAI Conference on Artificial Intelligence (AAAI’25), 2025 (Accepted) | ppt |
Demo Code: Github link
@inproceedings{lai2025pfedgpa, title={pFedGPA: Diffusion-based Generative Parameter Aggregation for Personalized Federated Learning}, author={Lai, Jiahao and Li, Jiagi and Xu, Jian and Wu, Yanru and Tang, Boshi and Chen, Siqi and Huang, Yongfeng and Ding, Wenbo and Li, Yang}, booktitle={Proceedings of the 38th Annual AAAI Conference on Artificial Intelligence (AAAI'25)}, year={2025}, note={Accepted} }