Multi-Modal Emotion Recognition: Extracting Public and Private Information
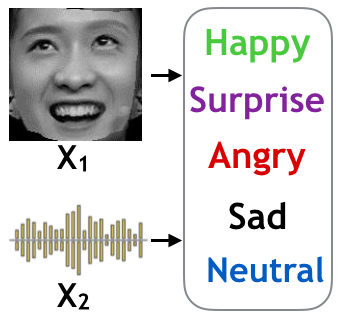
Multimodal emotion recognition is important for facilitating efficient interaction between humans and machines. To better detect emotional states from multimodal data, we need to effectively extract both the common information that captures dependencies among different modalities, and the private information that characterizes variations in each modality. However, existing works are mostly designed to pursue either one of these objectives but not both. In our work, we propose an end-to-end learning approach to simultaneously extract the common and private information for multimodal emotion recognition. Specifically, we use a correlation loss based on Hirschfeld-Gebelein-Renyi Maximal correlation and a reconstruction loss based on autoencoders to preserve the common and private information, respectively. Experimental results on eNTERFACE’05 database and RML database demonstrate the effectiveness of our proposed approach.
Publication
Ma, Fei, Wei Zhang, Yang Li, Shao-Lun Huang, and Lin Zhang. An end-to-end learning approach for multimodal emotion recognition: Extracting common and private information, In 2019 IEEE International Conference on Multimedia and Expo (ICME), pp. 1144-1149. IEEE, 2019. |
@inproceedings{ma2019end, title={An end-to-end learning approach for multimodal emotion recognition: Extracting common and private information}, author={Ma, Fei and Zhang, Wei and Li, Yang and Huang, Shao-Lun and Zhang, Lin}, booktitle={2019 IEEE International Conference on Multimedia and Expo (ICME)}, pages={1144--1149}, year={2019}, organization={IEEE} }