Finding the most transferable task for MRI brain segmentation
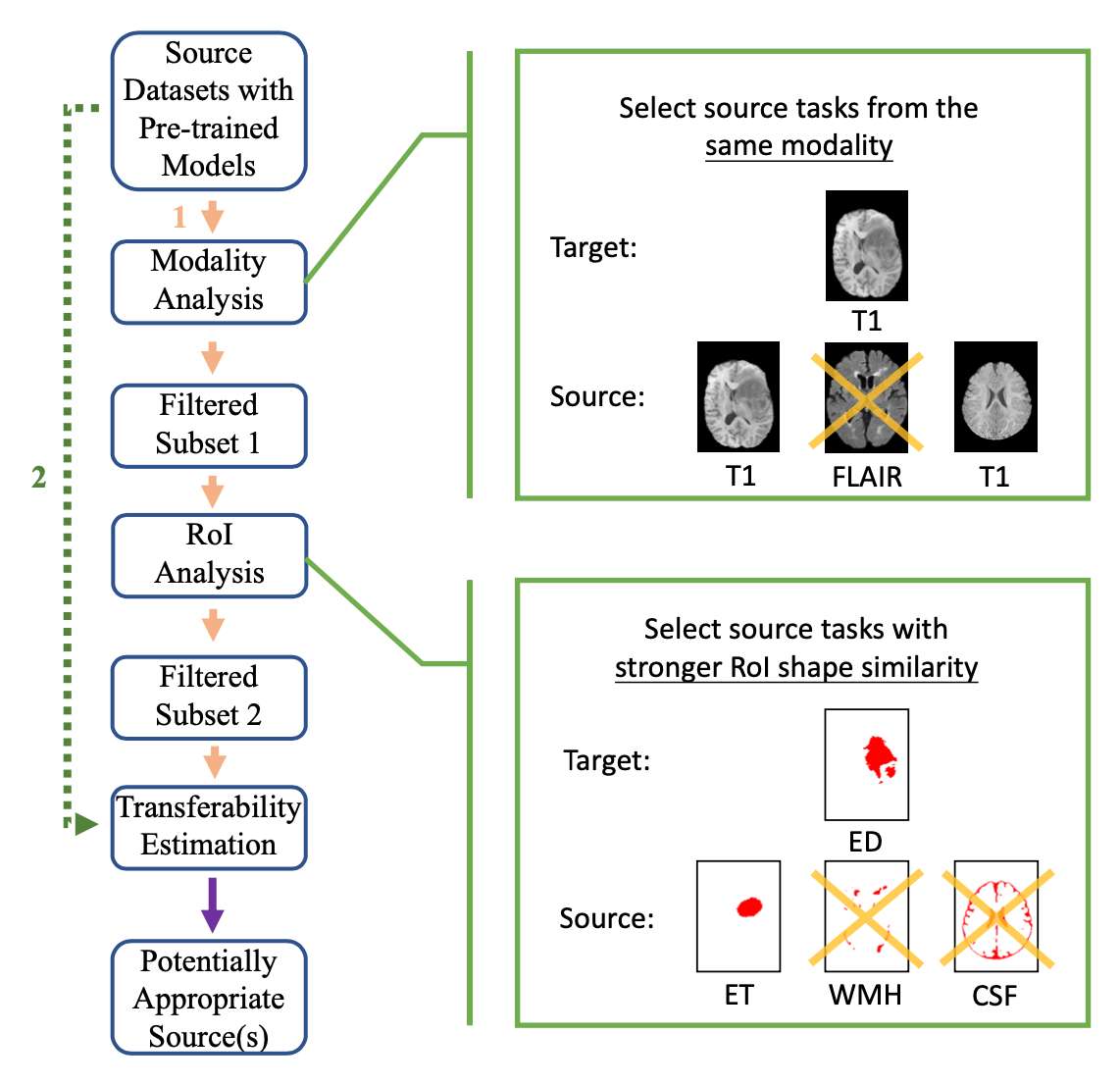
Although many studies have successfully applied transfer learning to medical image segmentation, very few of them have investigated the selection strategy when multiple source tasks are available for transfer. In this paper, we propose a prior knowledge guided and transferability based framework to select the best source tasks among a collection of brain image segmentation tasks, to improve the transfer learning performance on the given target task.
Specifically, our source selection framework refine the candidate source set in three steps: modality analysis, RoI (region of interest) analysis, and transferability estimation. It also adapts the state-of-the-art analytical transferability estimation metrics to medical image segmentation tasks and further show that their performance can be significantly boosted by filtering candidate source tasks based on modality and RoI characteristics.
Experiments on brain matter, brain tumor, and white matter hyperintensities segmentation datasets reveal that transferring from different tasks under the same modality is often more successful than transferring from the same task under different modalities. Furthermore, within the same modality, transferring from the source task that has stronger RoI shape similarity with the target task can significantly improve the final transfer performance. And such similarity can be captured using the Structural Similarity index in the label space.
Publication
Yicong Li, Yang Tan, Jingyun Yang, Yang Li*, and Xiao-Ping Zhang, Finding the Most TransferableTasks for Medical Image Segmentation, In Proceedings of the 2022 IEEE International Conference onBioinformatics and Biomedicine (BIBM), p.1620-1625, 2022 | ppt |
@inproceedings{li2022finding, title={Finding the Most Transferable Tasks for Brain Image Segmentation}, author={Li, Yicong and Tan, Yang and Yang, Jingyun and Li, Yang and Zhang, Xiao-Ping}, booktitle={2022 IEEE International Conference on Bioinformatics and Biomedicine (BIBM)}, pages={1620--1625}, year={2022}, organization={IEEE Computer Society} }