Enhancing Continuous Domain Adaptation with Multi-Path Transfer Curriculum
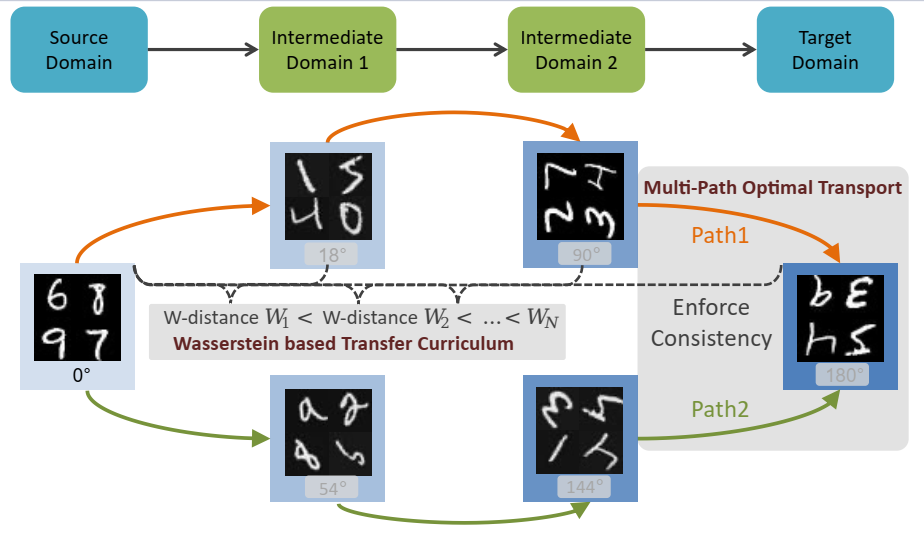
Addressing the large distribution gap between training and testing data has long been a challenge in machine learning, giving rise to fields such as transfer learning and domain adaptation. Recently, Continuous Domain Adaptation (CDA) has emerged as an effective technique, closing this gap by utilizing a series of intermediate domains. This paper contributes a novel CDA method, W-MPOT, which rigorously addresses the domain ordering and error accumulation problems overlooked by previous studies. Specifically, we construct a transfer curriculum over the source and intermediate domains based on Wasserstein distance, motivated by theoretical analysis of CDA. Then we transfer the source model to the target domain through multiple valid paths in the curriculum using a modified version of continuous optimal transport. A bidirectional path consistency constraint is introduced to mitigate the impact of accumulated mapping errors during continuous transfer. We extensively evaluate W-MPOT on multiple datasets, achieving up to 54.1% accuracy improvement on multi-session Alzheimer MR image classification and 94.7% MSE reduction on battery capacity estimation.
Publication
Hanbing Liu, Jingge Wang, Xuan Zhang, Ye Guo, and Yang Li. Enhancing Continuous Domain Adaptation with Multi-Path Transfer Curriculum. In Proceedings of The 28th Pacific-Asia Conference on Knowledge Discovery and Data Mining (PAKDD), Taipei, Taiwan, 2024 | ppt |
@inproceedings{liu2024enhancing, title={Enhancing Continuous Domain Adaptation with Multi-Path Transfer Curriculum}, author={Liu, Hanbing and Wang, Jingge and Zhang, Xuan and Guo, Ye and Li, Yang}, booktitle={Proceedings of the 28th Pacific-Asia Conference on Knowledge Discovery and Data Mining (PAKDD)}, year={2024}, address={Taipei, Taiwan}, month={May} }